Travel Demand Modeling
Understand the impact of individual behavior travel decisions, planning, and scheduling using our agent-based modeling tool, POLARIS.
Travel demand in POLARIS, Argonne’s high-fidelity predictive transportation system model, arises from a series of individual behaviors related to generating, planning, and scheduling routine and day-to-day activity engagements and travel. The interaction of the travel-demand model, which generates the activity engagement with the transportation supply model (LINK) that simulates trips, creates the network performance characteristics and travel outcomes that generate the metrics of interest when analyzing new technologies.
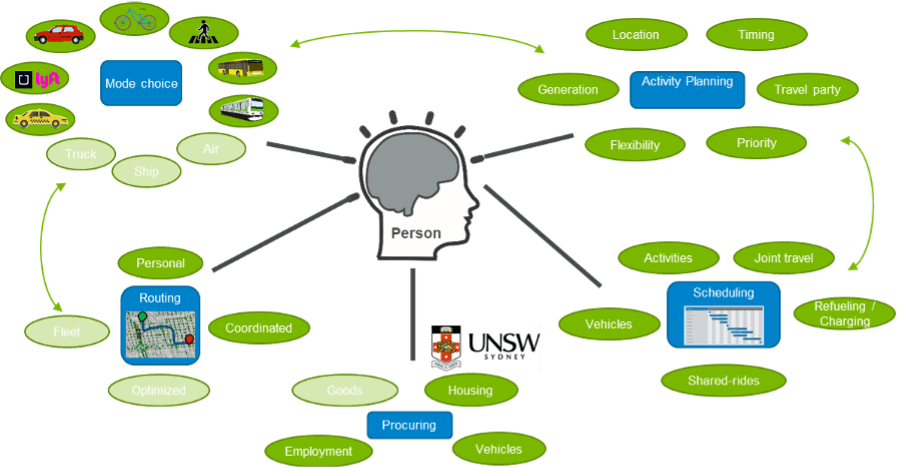
Developed by the Argonne National Laboratory Vehicle & Mobility Systems Group (VMS), POLARIS considers behavior across different timeframes, including:
- Long-term: in which behavior is impacted by household location/relocation, workplace choice, vehicle ownership, and technology choices, as well as other mobility options (e.g., bikes, transit passes, etc.);
- Mid-term: whereby work schedule, telecommuting, and household errands all have a significant influence; and
- Short-term: on a daily basis, traveler decisions are impacted by activity generation, planning (e.g., mode choice and location), scheduling (e.g., routing and conflict resolution), execution, and replanning.
POLARIS behavioral models are unique because they factor the travel demand model work in tandem with the long-term constraint, individual and household-level needs, and available travel options to develop a consistent daily activity and travel schedule for each agent. These are simulated on the model network as shown below:
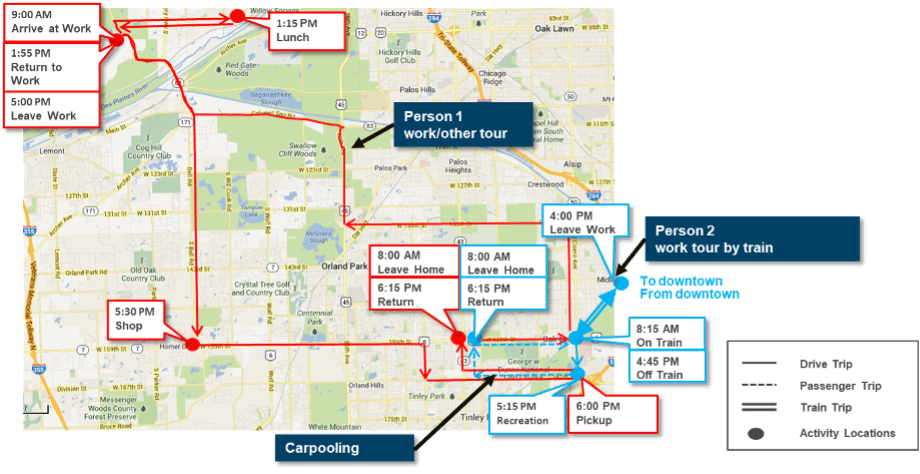
Key POLARIS travel demand features include:
- Cutting-edge statistical and behavior models used to represent most behaviors and decision making, including multinomial logit (MNL), nested logit (NL), mixed-logit, ordered-probit, individual choice and latent variable (ICLV), and other models; machine learning (e.g., decision trees, neural networks, heuristic rule-based models); and others;
- Estimates derived from a wide variety of behavior data from surveys, field data collection, sensors, etc.;
- Sensitivity to key levers relating to mobility and future vehicle technologies, including cost, travel times, value of time/reliability, accessibility, and many more; and
- Integration into a consistent modeling framework to represent dependencies between decisions using the POLARIS agent-based model architecture.